Large language models (LLMs) have demonstrated significant progress across various tasks, particularly in reasoning capabilities. However, effectively integrating reasoning processes with external search operations remains challenging, especially for multi-hop questions requiring intricate reasoning chains and multiple retrieval steps. Current methods primarily depend on manually designed prompts or heuristics, posing limitations in scalability and flexibility. Additionally, generating supervised data for multi-step reasoning scenarios is often prohibitively expensive and practically infeasible.
Researchers from Baichuan Inc., Tongji University, The University of Edinburgh, and Zhejiang University introduce ReSearch, a novel AI framework designed to train LLMs to integrate reasoning with search via reinforcement learning, notably without relying on supervised reasoning steps. The core methodology of ReSearch incorporates search operations directly into the reasoning chain. Utilizing Group Relative Policy Optimization (GRPO), a reinforcement learning technique, ReSearch guides LLMs to autonomously identify optimal moments and strategies for performing search operations, which subsequently influence ongoing reasoning. This approach enables models to progressively refine their reasoning and naturally facilitates advanced capabilities such as reflection and self-correction.

From a technical perspective, ReSearch employs structured output formats by embedding specific tags—such as <think>
, <search>
, <result>
, and <answer>
—within the reasoning chain. These tags facilitate clear communication between the model and the external retrieval environment, systematically organizing generated outputs. During training, ReSearch intentionally excludes retrieval results from loss computations to prevent model bias. Reward signals guiding the reinforcement learning process are based on straightforward criteria: accuracy assessment through F1 scores and adherence to the predefined structured output format. This design encourages the autonomous development of sophisticated reasoning patterns, circumventing the need for manually annotated reasoning datasets.
Experimental evaluation confirms the robustness of ReSearch. When assessed on multi-hop question-answering benchmarks, including HotpotQA, 2WikiMultiHopQA, MuSiQue, and Bamboogle, ReSearch consistently outperformed baseline methods. Specifically, ReSearch-Qwen-32B-Instruct achieved improvements ranging between 8.9% and 22.4% in performance compared to established baselines. Notably, these advancements were achieved despite the model being trained exclusively on a single dataset, underscoring its strong generalization capabilities. Further analyses demonstrated that models gradually increased their reliance on iterative search operations throughout training, indicative of enhanced reasoning proficiency. A detailed case study illustrated the model’s capacity to identify suboptimal search queries, reflect on its reasoning steps, and implement corrective actions autonomously.
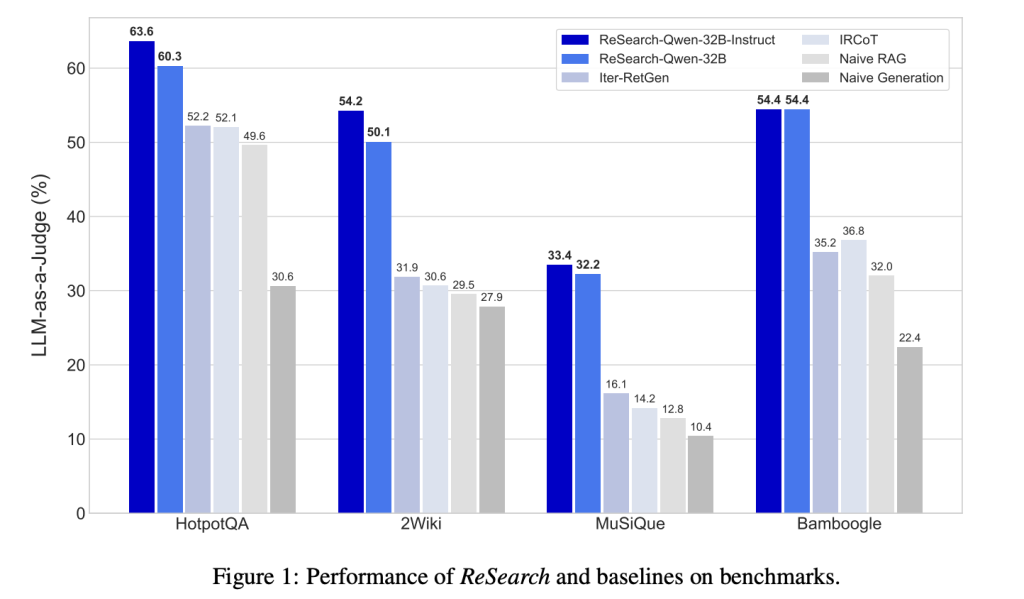
In summary, ReSearch presents a significant methodological advancement in training LLMs to seamlessly integrate reasoning with external search mechanisms via reinforcement learning. By eliminating dependency on supervised reasoning data, this framework effectively addresses critical scalability and adaptability issues inherent in multi-hop reasoning scenarios. Its capability for self-reflection and correction enhances its practical applicability in complex, realistic contexts. Future research directions may further extend this reinforcement learning-based framework to broader applications and incorporate additional external knowledge resources.
Check out the Paper and GitHub Page. All credit for this research goes to the researchers of this project. Also, feel free to follow us on Twitter and don’t forget to join our 85k+ ML SubReddit.
The post Meet ReSearch: A Novel AI Framework that Trains LLMs to Reason with Search via Reinforcement Learning without Using Any Supervised Data on Reasoning Steps appeared first on MarkTechPost.
Source: Read MoreÂ