A quote says, “Whoever owns the information owns the world.â€Â Â
In the digital era, having a solid hold on data is critical for companies that want to drive business success. Businesses should know how to utilize data strategically, more than owning a large volume of data. Not having a proper structure would cause disparities in data maturity levels, creating data silos and negatively affecting the organization’s business structure. When the day ends, data management comes down to only one thing: extracting information to make the best decisions possible. Â
Most companies are competing to find new ways to become data-driven, fast, and agile. Some want to jump straight from simple data storage to a fully functional predictive model. This can only be possible when they ensure superior data quality and security. And data maturity models (DMM) are pivotal in this. In this blog, we will talk about what data maturity models are, why they are important, and how you can achieve data maturity.Â
What is the Data Maturity Model (DMM), and Why is it Important?Â
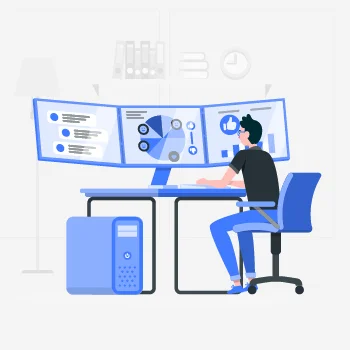
Before getting into DMM, let’s understand data maturity. It is a key measure of the health of an organization’s data resources. One can achieve optimal data maturity when all stakeholders effectively utilize quantitative and qualitative data. A data maturity model (DMM) allows businesses to assess their data governance practices, compare maturity levels with competitors, and communicate necessary improvements among stakeholders. Having optimal data maturity levels can help avoid poor data governance and management. Â
As a business owner, you may rely on luck and the natural growth of your company to develop data maturity, but it’s far-fetched and a bit of a gamble consisting of uncertainty and a time-consuming process. Instead, designing a data maturity model will help structure and streamline data maturity evolution. Let’s take a look at a few benefits that will explain by DMM is essential for businesses: Â
- Data maturity facilitates high-quality data and better decision-making, making it easier for managers to interpret and utilize the data they have researched and collected.Â
- Businesses gain a competitive benefit with the changes the data maturity model brings to the table and promote intelligent decision-making.Â
- Businesses can use DMM as a roadmap to identify their requirements and move to the next stage of data maturity.Â
- The data maturity model (DMM) allows businesses to integrate data analysis and automation within their operations seamlessly.Â
Core Components of a Data Maturity ModelÂ
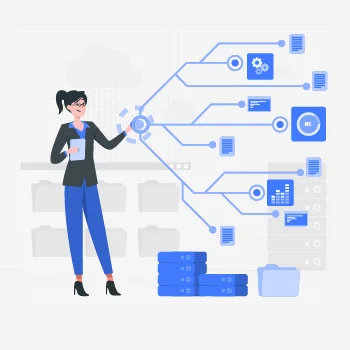
The data maturity model (DMM) allows organizations to assess their data management capabilities and identify areas for improvement. The core components include:Â
Components | Definition |
Data Governance | Businesses must establish data management roles, responsibilities, and policies to ensure compliance with data regulatory standards and internal policies. |
Data Quality | This component focuses on implementing data maintenance, cleansing, and validation processes to ensure data accuracy, reliability, and consistency. |
Data Architecture | It involves data modeling, integration, and infrastructure design to understand how data is stored, accessed, and organized.  |
Data Management Processes | This component focuses on data lifecycle management, process optimization, and standardization processes for collecting, using, and storing data. |
Data Security and Privacy | Businesses must ensure their data aligns with regulations like CCPA, HIPAA, GDPR, etc., protecting against breaches, unauthorized access, and loss. |
Data Analytics and Insights | It helps enable advanced analytics, visualization, and reporting to drive better decision-making with actionable data insights. |
Organizational Data Culture | Businesses should promote data literacy and collaboration within teams and implement data-driven decision-making across all levels. |
Technology and Tools | This involves leveraging appropriate tools for data integration, visualization, and analysis to support flexible and scalable solutions for business growth. |
Performance Measurement | Businesses should continuously monitor the progress of maturity goals and use KPIs to evaluate data management effectiveness.  |
5 Stages of a Data Maturity ModelÂ
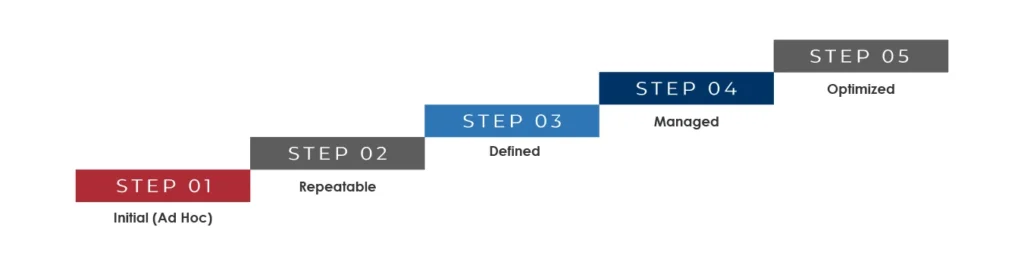
Understanding the five stages of data maturity is crucial to ensure that businesses become data-driven and progress. These stages enable organizations to assess their current approach, find loopholes, and implement improvement measures. Let’s have a look:Â
Stage 1: Initial (Ad Hoc)Â
The first stage involves identifying first-party data that is not structured and is siloed. There are no standardization processes for data management, and decisions are made without proper data support. Also, there are no proper KPIs for analyzing their impact.Â
Stage 2: RepeatableÂ
In this stage, businesses implement basic data management tools and processes, including data collection, storage, and reporting. The data is partially organized but still has inconsistencies, and teams need to document every process. Members receive fundamental analytics training and access to real-time problem-solving tools.Â
Stage 3: DefinedÂ
Data management strategies are formalized with clear governance standards and frameworks. This involves defining roles for data supervision and leveraging centralized data repositories, which will be the core of data-driven decision-making. Also, the processes are well documented and followed consistently.Â
Stage 4: ManagedÂ
This stage involves making every dataset count to extract maximum value from information resources. Businesses must consistently manage, integrate, and leverage data across their infrastructure. Data quality and accuracy are proactively monitored, and teams leverage advanced analytics and reporting mechanisms.Â
Stage 5 OptimizedÂ
This stage is the pinnacle of data maturity, and data becomes an asset that supports innovation, business growth, and CX. Businesses leverage ML and advanced analytics to extract insights from data and make better real-time decisions. Â
Types of Data Maturity ModelsÂ
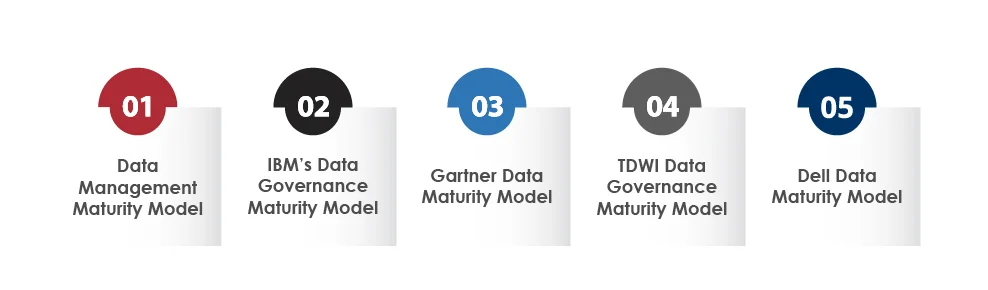
Some models are widely leveraged by organizations for their adaptability and effectiveness, which are listed below:Â
Data Management Maturity Model
This model provides a detailed framework for optimizing the data management process and ensuring consistency and repeatability across the enterprise’s levels. It mainly benefits organizations that want to formalize data management strategies and operations.Â
IBM’s Data Governance Maturity Model
This model makes data governance a critical component of data maturity. By leveraging this mode, businesses can easily align their data strategies with regulatory standards and compliance requirements.Â
Gartner Data Maturity Model
This model assists organizations in analyzing their data management process across five stages of data maturity. It offers a comprehensive overview of the data management process, from initial data collection to optimization and data-driven decision-making.Â
TDWI Data Governance Maturity Model
The model assists organizations understand the data governance maturity and decipher their assessment results. Businesses can use this model independently and can organize it into five dimensions: organization, architecture, resources, data life cycle, and governance.Â
Dell Data Maturity Model
This model offers an effective method to rank businesses based on their data maturity level. It uses a star system from one to four stars to rank a business. It involves four stages: Data Aware, Data Proficient, Data Savvy, and Data Driven.Â
Challenges in Achieving Data MaturityÂ
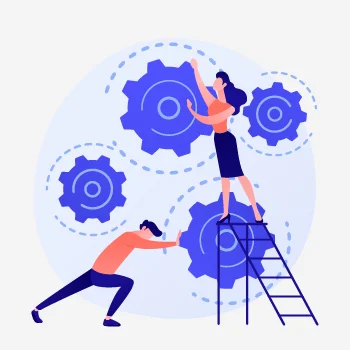
A survey shows that 87% of organizations have low data maturity levels. Organizations face various challenges throughout the journey of data maturity lifecycle. There are multiple factors, like unclear data strategies, poor data quality, fragmented silos, and culture and change challenges, which hinder consistent decision-making. Legacy systems and inadequate tools further complicate the governance and integration of increasing data volume. Additionally, regulatory compliance complexities and cost constraints are resource-intensive and contribute to slow data maturity. Tackling these challenges requires a strategic approach, partnering with professional data advisory firms like Tx, and investment in modern tech.Â
How Tx Data Advisory Can Help You Achieve Data Maturity?Â
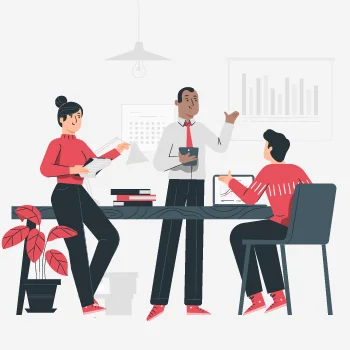
TestingXperts (Tx) offers comprehensive data advisory services, enabling clients to advance through the data maturity model. This helps enhance their data management capabilities and facilitate data-driven decision-making. Here’s how we can support you:Â
- We evaluate your existing data processes to identify gaps and areas for improvement. Our experts then design a comprehensive data strategy that aligns with your business goals and helps implement a data management foundation.Â
- We help implement standardized processes to ensure data consistency across the organization. We also introduce data validation and cleaning techniques to improve data reliability and accuracy.Â
- Our data experts help design and implement data governance frameworks to manage data assets effectively. This enables data consolidation into centralized repositories and eliminates silos for better access.Â
- We leverage advanced data analytics to help you gain deeper insights and support strategic decision-making. We ensure alignment with your business goals by establishing KPIs and metrics to monitor data management performance. Â
- Rating based on CMMI’s data maturity model to evaluate the current state of your data management function and staff capabilities.Â
SummaryÂ
High data maturity will result in more informed decisions, better business outcomes, and competitive benefits in the market. Therefore, as an organization, you must assess, grow, and optimize your data management practices and maturity models to enjoy the complete benefit of your data. The path from the initial to the optimized stage might be complex, but partnering with Tx would allow you to transform your management practices, leading to improved operational efficiency, sustained business growth, and informed decision-making. To know how Tx can help, contact our experts now.Â
FAQsÂ
Q1 What is the maturity model of data management?Â
The data management maturity model is a framework for helping organizations assess their data management capabilities and identify areas of improvement. It focuses on data governance, quality, integration, security, and analytics.Â
Q2 What is the purpose of the data maturity assessment?Â
A data maturity assessment helps in pinpointing gaps in an enterprise data governance framework and implementing clear policies, standards, and procedures to ensure data privacy and quality.Â
Q3 What are the five levels of the data maturity model (DMM)?Â
The five stages of the DMM are: initial, repeatable, defined, managed, and optimized. By following these stages, businesses can evaluate their data-related capabilities.Â
Q4 How Tx Data Advisory Can Help You Achieve Data Maturity?Â
TestingXperts data advisory services allow businesses to achieve data maturity by assessing current practices, implementing governance frameworks, and enhancing data integration capabilities. Tx experts ensure improved data accuracy, quality, scalability, and security.Â
The post Data Maturity Model: A Blueprint for Data-Driven Success first appeared on TestingXperts.
Source: Read More