MongoDB is excited to announce our integration with LangChainGo, making it easier to build Go applications powered by large language models (LLMs). This integration streamlines LLM-based application development by leveraging LangChainGo’s abstractions to simplify LLM orchestration, MongoDB’s vector database capabilities, and Go’s strengths as a performant, scalable, and easy-to-use production-ready language.
With robust support for retrieval-augmented generation (RAG) and AI agents, MongoDB enables efficient knowledge retrieval, contextual understanding, and real-time AI-driven workflows. Read on to learn more about this integration and the advantages of using MongoDB as a vector database for AI/ML applications in Go.
LangChainGo: Bringing LangChain to the Go ecosystem
LangChain is an open-source framework that simplifies building LLM-powered applications. It offers tools and abstractions to integrate LLMs with diverse data sources, APIs, and workflows, supporting use cases like chatbots, document processing, and autonomous agents. While LangChain currently supports only Python and JavaScript, the need for a similar solution in the Go ecosystem led to the development of LangChainGo.
LangChainGo is a community-driven, third-party port of the LangChain framework for the Go programming language. It allows Go developers to directly integrate LLMs into their Go applications, bringing the capabilities of the original LangChain framework into the Go ecosystem. LangChainGo enables users to embed data using various services, including OpenAI, Ollama, Mistral, and others. It also supports integration with a variety of vector stores, such as MongoDB.
MongoDB’s role as an operational and vector database
MongoDB excels as a unified data layer for AI applications with native vector search capabilities due to its simplicity, scalability, security, and rich set of features. With Atlas Vector Search built into the core database, there’s no need to sync operational and vector data separately—everything stays in one place, saving time and reducing complexity when you develop AI-powered applications. You can easily combine semantic searches with metadata filters, graph lookups, aggregation pipelines, and even geo-spatial or lexical search, enabling powerful hybrid queries all within a single platform.
MongoDB’s distributed architecture allows the usage of vector search to scale independently from the core database, ensuring optimized vector query performance and workload isolation for superior scalability. Plus, with enterprise-grade security and high availability, MongoDB provides the reliability and peace of mind you need to power your AI-driven applications at scale.
MongoDB, Go, and AI/ML
As the Go AI/ML landscape grows, MongoDB continues to drive innovation with its powerful vector search capabilities and LangChainGo integration, empowering developers to build RAG implementations and AI agents. This integration is powered by the MongoDB Go Driver, which supports vector search and allows developers to interact with MongoDB directly from their Go applications, streamlining development and reducing friction.
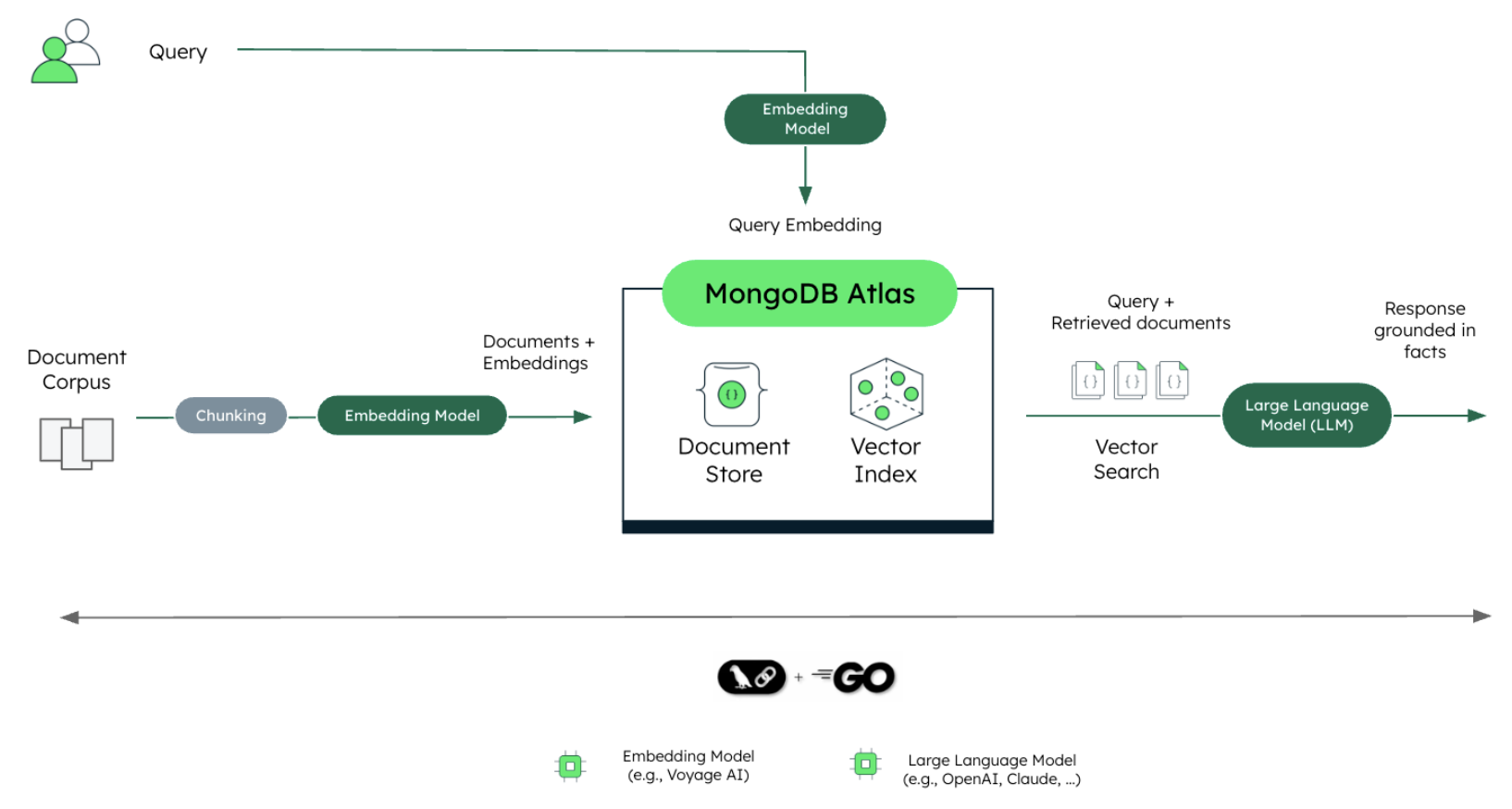
While Python and JavaScript dominate the AI/ML ecosystem, Go’s AI/ML ecosystem is still emerging—yet its potential is undeniable. Go’s simplicity, scalability, runtime safety, concurrency, and single-binary deployment make it an ideal production-ready language for AI. With MongoDB’s powerful database and helpful learning resources, developers can seamlessly build next-generation AI solutions in Go. Ready to dive in? Explore the tutorials below to get started!
Getting Started with MongoDB and LangChainGo
MongoDB was added as a vector store in LangChainGo’s v0.1.13 release. It is packaged as mongovector, a component that enables developers to use MongoDB as a powerful vector store in LangChainGo. Usage guidance is provided through the mongovector-vectorstore-example, along with the in-depth tutorials linked below. Dive into this integration to unlock the full potential of Go AI applications with MongoDB.
We’re excited for you to work with LangChainGo. Here are some tutorials to help you get started:
-
Retrieval-Augmented Generation (RAG) with Atlas Vector Search
-
Get started with Atlas Vector Search (select Go from the dropdown menu)
Source: Read More