- What is Predictive Data Analytics?
- Types of Predictive Data Analytics
- How does Predictive Analytics Optimize QA and Testing?
- 3 Reasons to Consider Predictive Analytics for Software Testing
- Leveraging AI and ML in Predictive Analytics to Upscale QA
- Why Partner with TestingXperts?
- Summary
Data is the core of business decision-making, and predictive data analytics is one significant solution that supports this process. Predictive analytics, a subset of data analytics, gained popularity for its capabilities to predict business outcomes, identify risks, suggest mitigation measures, and assist businesses in profit generation. In 2023, the predictive analytics industry was valued at $14.9 billion, expected to reach $41.44 billion by 2028. Given its rapid growth, this solution will be critical in driving business success. Â
But what role does predictive analytics play in quality assurance? How will it transform the QA process? Why should businesses integrate predictive analytics into their testing processes? Let’s find out.Â
What is Predictive Data Analytics?
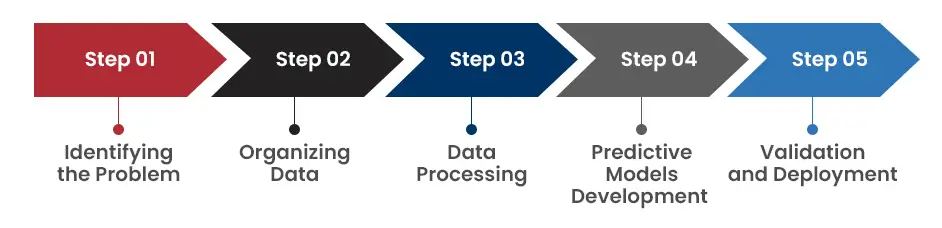
Predictive analytics leverage historical data and analytics techniques like ML, data mining, and statistical modeling to predict future outcomes/insights with notable accuracy. The process involves using diverse data sources, such as web scrapers, to extract and consolidate large datasets. Organizations integrate advanced analytics with these datasets to gain valuable insights. Advancements in Big Data ML also played a crucial role in further enhancing predictive analytics capabilities. Â
How does it work?Â
Data scientists and engineers leverage predictive models to identify similarities between multiple elements within datasets. After collecting data, they develop a statistical model, which they further train and modify to generate accurate predictions. The workflow involves the following steps:Â
Step 1: Identifying the ProblemÂ
A good prediction begins with a detailed set of requirements. For example, do you want to identify fraud using predictive analytics? What could be an ideal inventory level during the peak shopping season?Â
Step 2: Organizing DataÂ
Businesses generally have an abundance of data to work on. They should identify the data flow and organize datasets in a repository before developing predictive analytics models. Â
Step 3: Data ProcessingÂ
The next step is to clean the data by removing anomalies, extreme outliers, and missing data points resulting from measurement or input errors and preparing it for predictive analytics models. Â
Step 4: Predictive Models DevelopmentÂ
Data scientists and engineers leverage various techniques and tools to develop predictive models. It totally depends upon the dataset’s nature and the problem that needs resolution. ML, decision trees, Regression models, etc., are some common predictive model types that organizations use.Â
Step 5: Validation and DeploymentÂ
Check model accuracy and adjust accordingly. After achieving the desired outcomes, make them available to stakeholders via a data dashboard, app, or website.Â
Types of Predictive Data Analytics
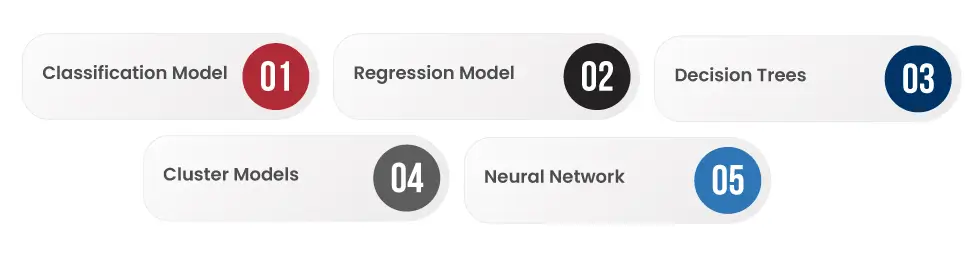
Classification ModelÂ
This model uses past data to create algorithms for categorizing datasets into multiple classes. For example, banks can use classification models to predict whether a borrower is in a high-risk or low-risk category, enabling informed lending decisions. Â
Regression ModelÂ
This model can only analyze quantitative variables. For example, a digital marketing agency can leverage past data to analyze a user’s average time watching a particular ad. The standard regression models used to understand the relation between variables are linear, logistic, and multiple regression. Â
Decision TreesÂ
With its simplicity and interpretability capabilities, a decision tree visually represents the potential outcomes. Depending on varying decisions, it consists of a root node and multiple internal nodes. The final outcomes are represented by leaf nodes, which means the tree is terminated. Businesses can use decision trees for both classification and regression tasks. Â
Cluster ModelsÂ
This model uses clustering to group similar data types (called clusters) and identify patterns and trends within them. Businesses can gain valuable insights by analyzing these clusters and identifying the factors responsible for similarities.Â
Neural NetworkÂ
This is one of the complex predictive analytics techniques similar to the human brain’s pattern recognition mechanisms. It effectively verifies regression model results and identifies non-linear relationships in large datasets. A neural network consists of an input layer, multiple computational layers, and an output layer to process complex data and generate relevant predictions.
How do Predictive Analytics Optimize Quality Assurance and Testing?
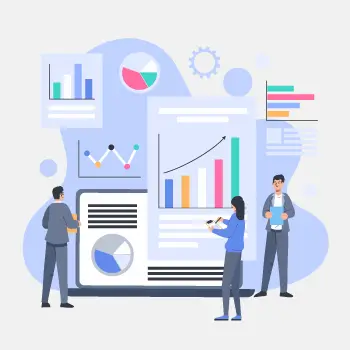
Predictive data analytics has transformed the QA process by allowing the teams to forecast potential software functionality issues and remain more proactive instead of reactive. This data-driven capability will enable businesses to predict problems faster, which helps them to stay proactive and plan mitigation measures early. Predictive analytics leverages multiple algorithms to understand past and current data gathered from testing tools. The algorithms then detect and analyze patterns humans miss, enabling businesses and their QA teams to identify and remediate loopholes early. Â
3 Reasons to Consider Predictive Analytics for Software Testing
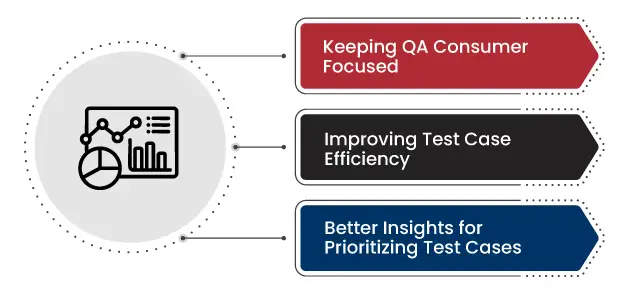
Faster time to market, consistency, and staying accurate are the primary reasons for using predictive analytics in QA. Let’s take a look at some of the reasons to implement analytics in the QA and testing process:Â
Keeping QA Consumer Focused
Companies must understand market trends and users’ preferences to develop the right solutions for their target audience. Leveraging analytics in QA helps analyze user feedback on products and applications, making QA more consumer-focused. Businesses can also quickly address the application’s focus areas like performance, compatibility, or security issues. Predictive analytics allows companies to leverage customer feedback and deliver quality solutions for better CX.
Improving Test Case Efficiency
QA teams work with multiple testing tools, monitor log files, and generate test scripts to deliver relevant solutions. Predictive analytics facilitates early detection of potential defects/failures by enabling a shift-left approach in testing. It can boost the QA process, enabling teams to take preventive actions beforehand and mitigate potential threats from the application.Â
Better Insights for Prioritizing Test Cases
Software development and testing activities generate massive data that must be effectively managed for further optimization. QA teams use various tools to analyze the data for defect logs, test cases and their results, project documentation, application log, and other QA files. Predictive Analytics can help examine testing and production environment defects, evaluate CX levels, identify issue patterns, and more. Businesses can use this data to prioritize test cases for higher coverage and QA process optimization.Â
Leveraging AI and ML in Predictive Analytics to Upscale QAÂ
Cognitive automation, powered by AI and ML, can handle high-level tasks that require human expertise. For example, in predictive analytics, AI/ML-powered cognitive automation transforms how businesses develop, test, and deploy their services/products to meet user expectations. AI and ML help make intelligent and accurate predictions about whether the software has defects. Â
AI and ML enable businesses to perform predictive analytics through cognitive automation in the quality assurance process. They can train their models by feeding quality data, which can later be used to predict future data, such as whether there will be a defect in the software or the build’s success.Â
Â
Why Partner with TestingXperts?
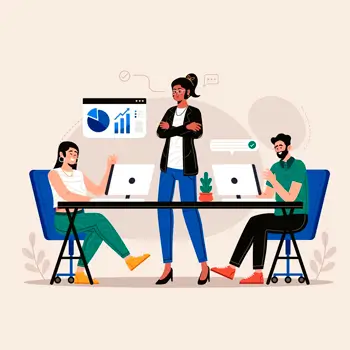
We at Tx perform robust QA testing to ensure the accuracy of your predictive models and deliver accurate results. By flagging errors early, you will get reliable insights for better decision-making. Also, by leveraging predictive analytics in software testing, we use previous performance metrics to predict future outcomes with almost 100% accuracy. This helps you cut QA costs by reducing repetitive test cases and identifying errors sooner. Our team possesses expertise in various AI and ML techniques, ensuring adaptability to diverse business applications. Our tailored approach addresses our clients’ unique QA challenges and business objectives. By leveraging our AI-powered in-house accelerators (Tx-Automate, Tx-SmarTest, etc.), we ensure the complete coverage of test cases to address the complexities of the QA process. Â
Summary
Predictive data analytics helps businesses tackle complex challenges more efficiently. By integrating historical data with statistical methods, predictive analytics assists businesses in forecasting future results to make informed decisions. However, data is a critical factor in facilitating this whole process. Enterprises rely heavily on data to strategize and plan their business goals. Effectively collecting, analyzing, and interpreting data offers detailed insights into market trends, potential growth areas, and operational efficiencies. Similarly, the quality assurance process requires data points from its development and testing repository to make intelligent decisions for testing applications. Contact our data testing and analytics experts to learn how Tx can help you drive business value from massive test data.Â
The post How Predictive Data Analytics Transforms Quality Assurance first appeared on TestingXperts.
Source: Read More